Computational Electronic Structure Theory (CEST)
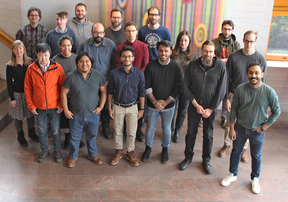
Research
We develop density-functional theory (DFT) for the electronic ground state and Green's function methods for excited states. Our favourite Green's function method is the GW approach. We are currently researching the application of GW to core level spectroscopy, a powerful tool to characterize molecules, liquids and adsorption processes at surfaces. We are also going beyond GW by combining it with the configuration interaction method to capture static correlation in strongly correlated systems.
For wide dissemination, we implement most of our developments into the Fritz Haber Institute ab initio molecular simulations package (FHI-aims). If you are interested in using FHI-aims for your own work or if you would like to contribute to FHI-aims, please contact us.
For more information on the GW approach, see our recent review article:
The GW Compendium: A Practical Guide to Theoretical Photoemission
Spectroscopy, D. Golze, M. Dvorak, and P. Rinke, Front. Chem. 7, 377
(2019)
For recent developments see:
Quantum embedding theory in the screened Coulomb interaction: Combining
configuration interaction with GW/BSE, M. Dvorak, D. Golze, and P.
Rinke, Phys. Rev. Materials 3, 070801(R) (2019)
Core-Level Binding Energies from GW: An Efficient Full-Frequency
Approach within a Localized Basis, D. Golze, J. Wilhelm, M. van Setten,
and P. Rinke, J. Chem. Theory Comput. 14, 4856 (2018)
Machine learning is a branch of artificial intelligence and is currently revolutionizing research practices in the natural sciences. Machine learning models are trained on materials data already available from experiments or computations by creating statistically optimized relationships between the given data. Once the model is trained sufficiently, it can make predictions for new materials or infer correlations with almost the same accuracy as the data generation method, but in only a fraction of the time and with a fraction of the computational or experimental effort. We currently pursue two main machine learning research lines: BOSS and ARTIST.
BOSS: Bayesian Optimization Structure Search is an active learning technique for global exploration of energy and property phase space, and for accelerated structure determination.
Bayesian inference of atomistic structure in functional materials, M. Todorović, M. U. Gutmann, J. Corander and P. Rinke, npj Comp. Mat. 5, 35(2019)
ARTIST: Artificial Intelligence for Spectroscopy is a suite of machine learning methods for excited states and spectral properties. We are exploring kernel ridge regression for individual excitation energies and neural networks for excitation spectra. We are also developing descriptors for atomistic representations. These descriptors are available in the DScribe Phython library.
Chemical diversity in molecular orbital energy predictions with kernel ridge regression, A. Stuke, M. Todorović, M. Rupp, C. Kunkel, K. Ghosh, L. Himanen, and P. Rinke, J. Chem. Phys. 150, 204121 (2019)
Deep Learning Spectroscopy: Neural Networks for Molecular Excitation Spectra, K. Ghosh, A. Stuke, M. Todorović, P. B. Jørgensen, M. N. Schmidt, A. Vehtari and P. Rinke, Adv. Sci. 6, 1801367 (2019)
DScribe: Library of descriptors for machine learning in materials science, L. Himanen, M. O. J. Jäger, E. V. Morooka, F. F. Canova, Y. S. Ranawat, D. Z. Gao, P. Rinke, and A. S. Foster, Comp. Phys. Commun. 247, 106949 (2020)
Data-driven science is heralded as a new paradigm in materials science. In this field, data is the new resource, and knowledge is extracted from materials datasets that are too big or complex for traditional human reasoning - typically with the intent to discover new or improved materials or materials phenomena. We are currently involved in the development of two materials infrastructures, the Novel Materials Discovery (NOMAD) laboratory, and the Aalto Materials Digitalization Platform.
Data‐Driven Materials Science: Status, Challenges, and Perspectives, L. Himanen, A. Geurts, A. S. Foster and P. Rinke, Adv. Sci. 6, 1900808 (2019)
Materials structure genealogy and high-throughput topological classification of surfaces and 2D materials, L. Himanen, P. Rinke, and A. S. Foster, npj Comput. Mater. 4, 52 (2018)
Biomaterials play a crucial role in our pursuit of a sustainable society. Feedstock from biomass (e.g., wood) processed in biorefineries can provide us with as a renewable source of materials such as chemicals, solvents, and polymers that can subsequently be incorporated into high-value products. Bio materials furthermore offer alternative routes for waste management through biodegradation processes and promote equality in the global economy by decreasing our reliance on scarce raw materials.
To accelerate the development of new technologies for biomaterials, we are researching machine learning-assisted approaches to materials processing and modelling. Our current efforts focus on applying Bayesian optimization, through our in-house developed code BOSS, as a means of planning experiments and predicting their outcome.
For an application of machine learning and BOSS to optimize a novel biorefinery concept for green lignin extraction based on hydrothermal pre-treatment of hardwood followed by aqueous-acetone extraction see:
For an application of machine learning and BOSS to predict the morphology of colloidal, oxidized tannic acid particles see:
Molecules may aggregate into aerosols in the atmosphere. Such cluster formation affects air quality and the climate. We develop and apply artificial intelligence (AI) methods to model molecular processes in the atmosphere and to predict and understand molecular cluster formation. We are also developing digital twins of molecular processes and scientific instruments for a virtual laboratory in atmospheric science. The CEST group is part of the Virtual Laboratory for Molecular Level Atmospheric Transformations (VILMA) Centre of Excellence.
Predicting gas–particle partitioning coefficients of atmospheric molecules with machine learning, E. Lumiaro, M. Todorović, T. Kurten, H. Vehkamäki, and P. Rinke, Atmos. Chem. Phys. 21, 13227 (2021)
Clean energy use and generation is one of society's grandest challenges. We apply our electronic structure theory and machine learning methods to study suitable materials for clean energy solutions. We investigate novel hybrid perovskite solar cell materials, catalysts for hydrogen production and organic light emitters and solar cells.
Database-driven high-throughput study of coating materials for hybrid perovskites, A. Seidu, L. Himanen, J. Li, and P. Rinke, New J. Phys. 21,
083018 (2019)
Activation Energy of Organic Cation Rotation in CH3NH3PbI3 and CD3NH3PbI3: Quasi-Elastic Neutron Scattering Measurements and First-Principles Analysis Including Nuclear Quantum Effects, J. Li, M. Bouchard, P. Reiss, D. Aldakov, S. Pouget, R. Demadrille, C. Aumaitre, B. Frick, D. Djurado, M. Rossi, and P. Rinke, J. Phys. Chem. Lett. 9, 3969 (2018)
Research into future technologies has come to focus on miniature multi-material devices and nanostructures, with the intent of harnessing quantum mechanical phenomena to perform an automated function such as generating signals or separating atoms or charges. Organic and inorganic materials are frequently employed side-by-side to take advantage of their unique capabilities. While the properties of the individual substances or bulk materials are known, it is not always possible to predict or measure what occurs at the boundary between them.
We apply our electronic structure theory and machine learning methods to organic-molecule protected noble metal clusters, DNA-stabilized silver clusters, and organic films on inorganic semiconductors and metals. These systems hold great potential for applications in electronic devices, catalysis, biochemical sensing and medical treatments.
Bayesian inference of atomistic structure in functional materials, M. Todorović, M. U. Gutmann, J. Corander and P. Rinke, npj Comp. Mat. 5, 35(2019)
Optical Properties of Silver-Mediated DNA from Molecular Dynamics and Time Dependent Density Functional Theory, E. Makkonen, P. Rinke, O. Lopez-Acevedo, and X. Chen, Int. J. Mol. Sci. 19, 2346 (2018)
Charge-transfer driven nonplanar adsorption of F4TCNQ molecules on epitaxial graphene, A. Kumar, K. Banerjee. M. Dvorak. F. Schulz. A. Harju, P. Rinke and P. Liljeroth, ACS Nano 11, 4960 (2017)
Latest Publications
Validation of the GreenX library time-frequency component for efficient GW and RPA calculations
Benchmarking the accuracy of the separable resolution of the identity approach for correlated methods in the numeric atom-centered orbitals framework
Machine-learning accelerated structure search for ligand-protected clusters
Tailoring Hot-Carrier Distributions of Plasmonic Nanostructures through Surface Alloying
Using scalable computer vision to automate high-throughput semiconductor characterization
Time-frequency component of the GreenX library: minimax grids for efficient RPA and GW calculations
Atomic structures, conformers and thermodynamic properties of 32k atmospheric molecules
Ferrofluidic Manipulator : Theoretical Model for Single-Particle Velocity
Core-Selective Silver-Doping of Gold Nanoclusters by Surface-Bound Sulphates on Colloidal Templates: From Synthetic Mechanism to Relaxation Dynamics
Gold Au(I)6 Clusters with Ligand-Derived Atomic Steric Locking: Multifunctional Optoelectrical Properties and Quantum Coherence
Aalto Materials Digitalization Platform - AMAD
Materials data infrastructure for Aalto University
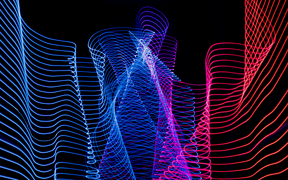
Research Group Members
Group Leader
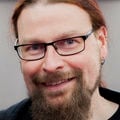
Lecturers
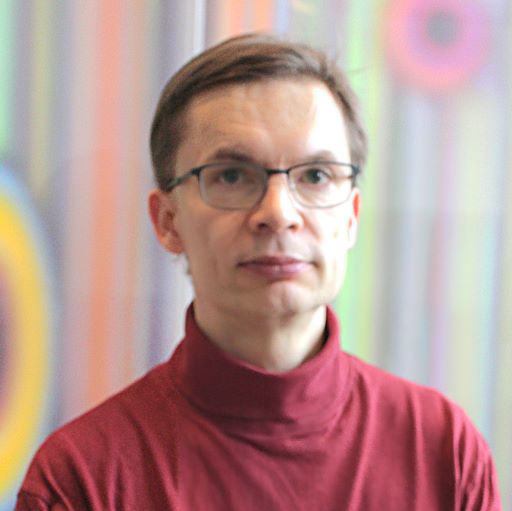
Postdoctoral Researchers
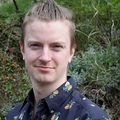
Armi Tiihonen
Doctoral, Master Students and Research Assistants
Lucas Bandeira
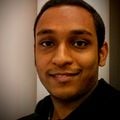
Kunal Ghosh
Henrietta Homm
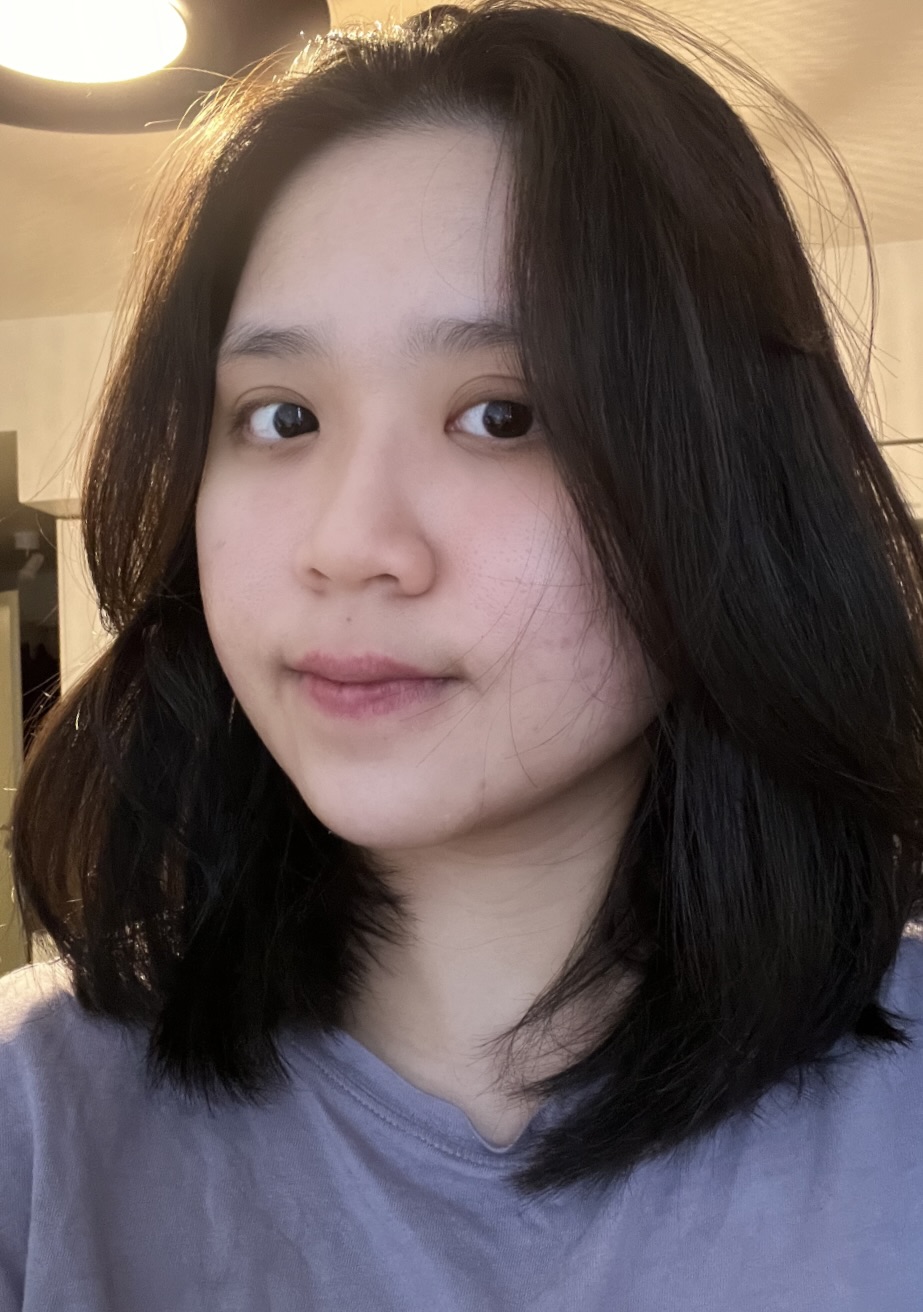
Tatu Linnala
Visiting Researchers
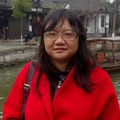
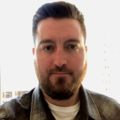
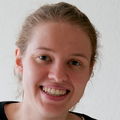
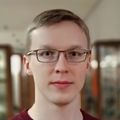
Matthias Stosiek
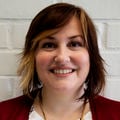
News
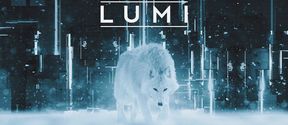
CEST researchers receive significant LUMI supercomputing resources
Read how two successful machine learning projects got support by a supercomputer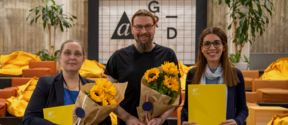
Aalto Open Science Award ceremony brought together Aaltonians to discuss open science
Last week we gathered at A Grid to celebrate the awardees of the Aalto Open Science Award 2023 and discuss open science matters with the Aalto community.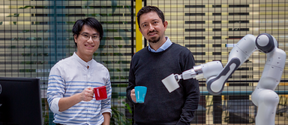
Aalto Open Science Award Third Place Awardee 2023 – Intelligent Robotics Research Group with the Robotic Manipulation of Deformable Objects project
We interviewed the Intelligent Robotics Research Group with the Robotic Manipulation of Deformable Objects project, 3rd place awardees of the first Aalto Open Science Award.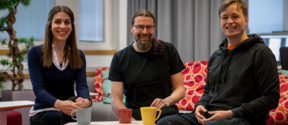