Defence of doctoral thesis in the field of computer science, Ari Heljakka
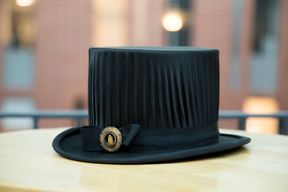
When
Where
Event language(s)
Title of the doctoral thesis is "Deep Generative Neural Network Models for Capturing Complex Patterns in Visual Data"
Generative neural networks allow one to synthesize and semantically edit complex data structures such as images or objects. In recent years, GAN models have excelled in producing realistic imagery. However, typically, these models are limited to producing random images from a given category. Instead, one would often like to edit existing content and combine attributes of suitable exemplar images. This requires encoding the images into a compact form the model can understand. Generative autoencoders are models that could serve the purpose, but they tend to produce blurry images, even at low resolutions.
In this dissertation, autoencoders and other machine learning methods were developed to resolve these issues. The models allow for exemplar-based sharp editing of existing high-resolution images at the level of semantic attributes, such as face expression or jaw shape. It was shown that, contrary to the general belief, autoencoders can in fact synthesize images realistic enough to make it hard for humans to tell them apart from real ones. Hence, the gap between autoencoders and GANs has narrowed down, without losing the autoencoder ability to instantaneously process and combine the attributes of user-provided images. The model learns completely unsupervised, with no labeled data. Finally, the user can choose to add a desired proportion of random variation into the synthesized images, in a GAN-like fashion.
Ideally, based on the training data of the relevant subdomain of science or engineering, a generative model learns the “design landscape” of physically acceptable and desirable solutions. In the new ensuing paradigm, the task of the human designer will be, then, to choose the high-level attributes of images or other data structures in desired proportions. The AI will then combine them in a realistic manner.
Opponent: Professor Ole Winther, Technical University of Denmark DTU, Denmark
Custos: Professor Juho Kannala, Aalto University School of Science, Department of Computer Science
Contact details of the doctoral candidate: Ari Heljakka, Department of Computer Science, p. +358 50 428 0606, [email protected]
The defence will be organized via remote technology (Zoom). Link to the defence
The doctoral thesis is publicly displayed 10 days before the defence in the publication archive of Aalto University (aaltodoc.aalto.fi).
- Published:
- Updated: