You can’t tell whether an online restaurant review is fake—but this AI can

Sites like TripAdvisor, Yelp and Amazon display user reviews of products and services. Consumers take heed: nine out of ten people read these peer reviews and trust what they see. In fact, up to 40% of users decide to make a purchase based on only a couple of reviews, and great reviews make people spend 30% more on their purchases.
Yet not all reviews are legitimate. Fake reviews written by real people are already common on review sites, but the amount of fakes generated by machines is likely to increase substantially.
According to doctoral student Mika Juuti at Aalto University, fake reviews based on algorithms are nowadays easy, accurate and fast to generate. Most of the time, people are unable to tell the difference between genuine and machine-generated fake reviews.
‘Misbehaving companies can either try to boost their sales by creating a positive brand image artificially or by generating fake negative reviews about a competitor. The motivation is, of course, money: online reviews are a big business for travel destinations, hotels, service providers and consumer products,’ says Mika Juuti.
In 2017, researchers from the University of Chicago described a method for training a machine learning model, a deep neural network, using a dataset of three million real restaurant ratings on Yelp. After the training, the model generated fake restaurant reviews character by character.
There was a slight hiccup in the method, however; it had a hard time staying on topic. For a review of a Japanese restaurant in Las Vegas, the model could make references to an Italian restaurant in Baltimore. These kinds of errors are, of course, easily spotted by readers.
To help the review generator stay on the mark, Juuti and his team used a technique called neural machine translation to give the model a sense of context. Using a text sequence of ‘review rating, restaurant name, city, state, and food tags’, they started to obtain believable results.
‘In the user study we conducted, we showed participants real reviews written by humans and fake machine-generated reviews and asked them to identify the fakes. Up to 60% of the fake reviews were mistakenly thought to be real,’ says Juuti.
Juuti and his colleagues then devised a classifier that would be able to spot the fakes. The classifier turned out to perform well, particularly in cases where human evaluators had the most difficulties in telling whether a review is real or not.
The study was conducted in collaboration with Aalto University’s Secure Systems research group and researchers from Waseda University in Japan. It was presented at the 2018 European Symposium on Research in Computer Security in September.
The work is part of an ongoing project called Deception Detection via Text Analysis in the Secure Systems group at Aalto University.
Research articles:
Mika Juuti, Bo Sun, Tatsuya Mori, N. Asokan:
Stay On-Topic: Generating Context-specific Fake Restaurant Reviews. https://arxiv.org/abs/1805.02400
More information:
Mika Juuti, Doctoral Candidate
Aalto University
Secure Systems group
[email protected]
tel. +358 50 560 7944
N. Asokan, Professor
Aalto University
Secure Systems group
[email protected]
tel. +358 50 483 6465
- Published:
- Updated:
Read more news

Viktar Asadchy receives Young Scientist Award
The Finnish Foundation for Technology Promotion awarded Assistant Professor Viktar Asadchy with the Young Scientist 2024 Award.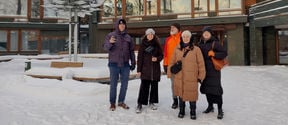
Preserving intangible cultural heritage through immersive XR experiences
Aalto University’s Department of Art and Media is coordinating a European wide project on preserving intangible cultural heritage and using it to address societal challenges with the help of immersive XR environments.