Machine Learning for Big Data
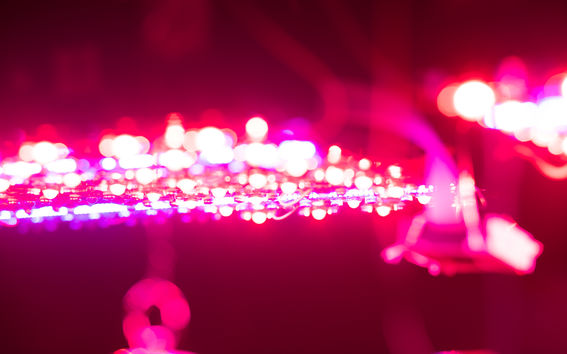
Research
Our research revolves around machine learning models and methods for big data over networks. The data arising in many important big data applications, ranging from social networks to network medicine, consist of high-dimensional data points related by an intrinsic (complex) network structure. In order to jointly leverage the information conveyed in the network structure as well as the statistical power contained in high-dimensional data points, we study networked exponential families. For the accurate learning of such networked exponential families, we borrow statistical strength, via the intrinsic network structure, across the dataset. A powerful algorithmic toolbox for designing learning algorithms is provided by convex optimization methods. Modern convex optimization methods are appealing for big data applications as they can be implemented as highly scalable message passing protocols.
Latest publications
Analysis of Total Variation Minimization for Clustered Federated Learning
Maschinelles Lernen: Die Grundlagen
The Aalto Dictionary of Machine Learning
Engineering Trustworthy AI : A Developer Guide for Empirical Risk Minimization
From intangible to tangible : The role of big data and machine learning in walkability studies
Explainable empirical risk minimization
Hercules
A perspective on the enabling technologies of explainable AI-based industrial packetized energy management
Towards Model-Agnostic Federated Learning over Networks
Moreau Envelope ADMM for Decentralized Weakly Convex Optimization
People
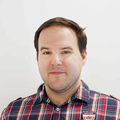
Henrik Ambos
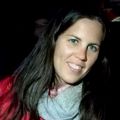
Laia Amorós Carafí
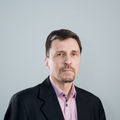
Timo Huuhtanen
Arttu Mäkinen
Roope Tervo
Nguyen Tran
- Published:
- Updated: