Machine learning helps design new materials

Most regular objects tend to stretch ‘evenly’, that is: scientists can predict how much force is required to make a material stretch by a certain distance. Recent experiments have shown that these predictions don’t hold up at the micrometre scale. The stretching of microscopic crystals happens in discrete bursts with a very wide size distribution. Since the bursts occur sporadically, seemingly identical micro-scale samples can stretch in very different ways. This variability of the strength characteristics of the samples poses a challenge for the development of novel materials with desired properties. In their article Machine learning plastic deformation of crystals published in Nature Communications, the researchers use machine learning to predict the characteristics of individual samples.
'The machine learning algorithms succeeded in measuring how predictable the stretch process of small crystalline samples is. This would have been practically impossible with traditional means, but machine learning enables the discovery of new and interesting results,' explains Associate Professor Lasse Laurson from the Laboratory of Physics at Tampere University of Technology.
The irreversible plastic deformation of crystalline substances occurs when crystallographic defects, called dislocations, move from one location in the crystal to another. Crystalline materials, such as metals or ice almost always contain networks of dislocations, with each crystal containing its own network.
The researchers trained machine learning algorithms to recognise the connection between an object’s microscopic structure and the amount of force required to stretch a sample. The study revealed, amongst other things, that the predictability of the amount of force required changes on the stretching of the sample: At first, it becomes harder to predict the force required as the stretch grows, which depends mostly on the stretch bursts’ sporadic nature. Surprisingly, however, predictability improves as the stretch continues to grow. Size also affects predictability: it is easier to predict the deformation process of larger crystals than smaller ones.
'As the stretch grows, the number of bursts reduces, consequently improving predictability. This is promising in terms of predicting the yield of individual samples, which is a key objective in material physics,' says Henri Salmenjoki, doctoral candidate at the Department of Applied Physics at Aalto University.
'Our research indicates that machine learning can be used to predict very complex and non-linear physical processes. In addition to the development of optimal materials, possible applications can be found in the prediction of dynamics of many other complex systems,' Laurson explains.
Professor Mikko Alava from Aalto University was also involved in the recently published study. The study received funding from the Academy of Finland.
Read more about the study in the Nature Communications publication.
- Published:
- Updated:
Read more news

Min-Kyu Paek has been appointed Assistant Professor at the Department of Chemical and Metallurgical Engineering
Min-Kyu Paek has been appointed Assistant Professor at the Department of Chemical and Metallurgical Engineering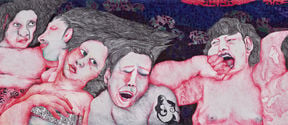
Aalto ARTS alum Vidha Samya’s artwork featured at the Venice Biennale 2024
The Pavilion of Finland presents ‘The pleasures we choose’ at the 60th International Art Exhibition – La Biennale di Venezia until 24 November 2024.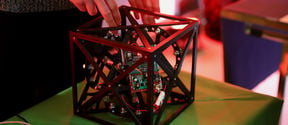
IoT Forge donates EUR 1 million to the School of Engineering
The donation will be used for research and education on the Industrial Internet and digital twins.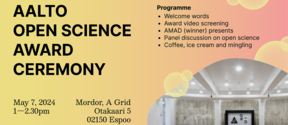