Reality Capture: Automated Construction Site Productivity and Quality Monitoring

Aalto’s Intelligent Construction Site (iCONS) project had demonstrated how process inefficiencies become visible when IoT sensors are used to track the positions and movements of workers and materials. However, researchers still had to interpret photos and videos to determine how the data correlated with the construction schedule, plans, and design models. The following project—Reality Capture—was conceived as an effort to investigate if, and how, the collection and interpretation of visual data could be automated.
The RECAP project was funded by Business Finland, Aalto University, and a consortium of five companies, including construction firms Fira and YIT and precast and ready-mix concrete manufacturer Rudus. The two IT providers were Vionice, a computer vision firm, and Umbra, a graphics software developer.
The project released its final report on January 30, 2020, with some interesting findings about the feasibility of current technologies in automating visual construction site monitoring.
The Challenges of Production and Quality Control in Construction
The RECAP research team started off with a round of interviews with the consortium companies and a selection of contractors from California, Brazil, and China.
All the interviewees were unanimous about the difficulties inherent in obtaining real-time and accurate data from a construction site. Consequently, managers struggle to keep workers engaged in the right tasks, at the right time. As a result, workers can rush into taking on unplanned tasks, which often results in incomplete work that has to finalized later in haste.
It seems to be common practice that both production and quality control are performed manually. In Brazil, specifically, quality inspection is costly and trained inspectors are in short supply. That, together with the reluctance to report quality issues, leads to excessive variation in quality.
The interviewed professionals agree that monitoring of progress and productivity require better solutions, especially for MEP installations. Furthermore, the comparison between what is planned and what has been built is still arduous. Some companies are trying out software that can automatically compare point clouds and building information models, but the technology is still in its infancy.
Automatic Detection of Work Progress
The project consortium chose five specific use cases. Three studies explored the possibilities for automated progress monitoring and two focused on quality inspections.
Fira’s two projects used photos that workers recorded during the plumbing renovation of a set of seven bathrooms and six kitchens. The purpose of the trial was to determine whether automated analysis of work progress would be possible using the photos. In such a case, the site manager could use the data to remotely monitor the progress and make necessary planning and management decisions accordingly.
The research team developed a progress inspection system that processed batches of photos associated with location and time data. The core of the system was a machine learning solution that used photos recorded using a tailor-made Android app. The system was designed to use pairs of consecutive photos from the construction site. The researchers were pleased to learn that the system was able to attain a good level of accuracy in determining the progress of construction work.
In the third project, YIT used a crane camera system developed by Pix4D. The system generates both 2D images and 3D point clouds of the construction site. The researchers used two alternative algorithms, VBUILT and MBUILD, for extracting buildings from 3D point clouds and in the case of MBUILD, aligning them with the BIM model.
Automated Quality Inspections
Another YIT project explored the feasibility of automated quality inspections of door moldings in a housing project. Workers made video recordings of each completed apartment, and an automated process determined—from the images—whether the quality of the moldings was acceptable. This proved to be a difficult task as there were not enough poor-quality examples to train the system properly. With more material, the inspection would be quite usable in real-life situations.
The fifth project took place in a precast staircase manufacturing plant of Rudus. The intention was to determine whether staircase rebars had been mounted as specified in the building information model. The researchers used a mini-PC and a camera attached to a bridge crane. The image data collected by this setup was sent to an Aalto server and laid over a preprocessed BIM model of the staircase. An inspector could scrutinize the model and reinforcement progress data via a web browser on Umbra’s cloud platform.
The researchers found that rebar detection was indeed feasible, but owing to the relatively small number of high-quality labelled images, it did not reach sufficient accuracy in this project.
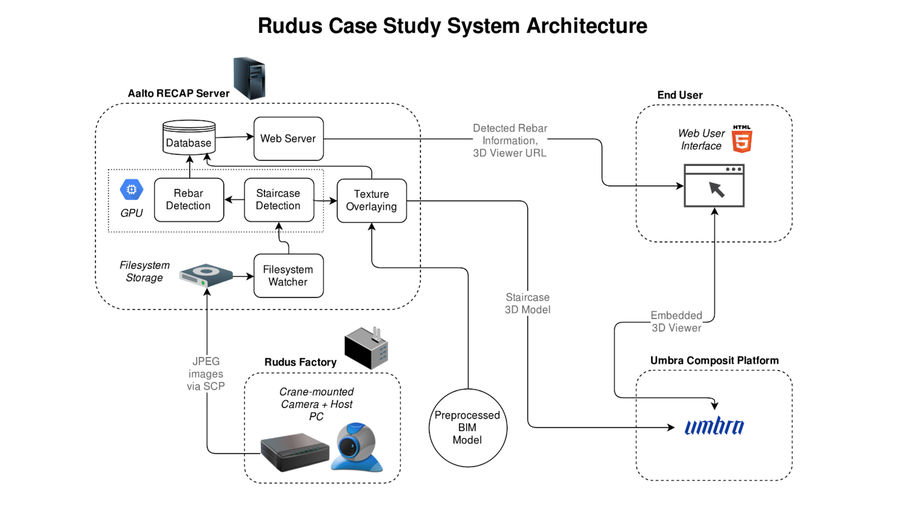
The Future of AR and AI in Construction Monitoring and Control
In addition to previously described pilot case studies, RECAP created an experimental augmented reality application for on-site use. The app offers a visual interface to cloud-based AI-powered processing of image data. It serves the three needs that contractors had determined as most critical when asked during the project: 1) to report on renovation progress and productivity monitoring, 2) to flag any defects, and 3) to share information with colleagues. The app heralds the kind of tools we’ll see more of in the future on construction sites.

The researchers involved in the Reality Capture project concluded that it is technically feasible to use AI, point clouds, and images in construction progress monitoring and control. Still, they considered data collection as the bottleneck in the process. Without sufficient data, learning algorithms will not be sufficiently accurate for large-scale use.
360-degree helmet-mounted cameras will possibly come to the rescue, but even they require a lot of manual labelling work. Commercial implementations are easiest in repetitive interiors—for example, in hotel rooms, bathrooms, and kitchens.
The use of crane cameras currently offers the most promising technology for detecting work progress on a construction site. Moreover, if BIM models are available, the technique becomes even more accurate.
In summary, machine vision and deep learning methods require data that is not yet readily available. If data collection and its post-processing can be automated, the construction industry will take a big step toward a more industrialized production system.
Download the Reality Capture (RECAP) project final reportby Olli Seppänen, Yu Xiao, Mustafa Khalid Masood, Petr Byshev, Truong-An Pham, Antti Aikala, Pontus Lundström.
- Published:
- Updated:
Read more news

Viktar Asadchy receives Young Scientist Award
The Finnish Foundation for Technology Promotion awarded Assistant Professor Viktar Asadchy with the Young Scientist 2024 Award.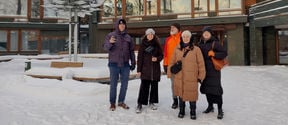
Preserving intangible cultural heritage through immersive XR experiences
Aalto University’s Department of Art and Media is coordinating a European wide project on preserving intangible cultural heritage and using it to address societal challenges with the help of immersive XR environments.