How machine learning can support atmospheric compound discovery

The identification of chemical compounds found in the atmosphere is challenging and tedious, currently relying on mass spectrometry measurements. A new perspective paper is now discussing what promise machine learning holds to accelerate and improve the accuracy of ongoing studies aimed at mapping new atmospheric compounds. Such compounds are worthwhile studying as they contribute to atmospheric particle formation, therefore directly impacting climate as well as air quality.
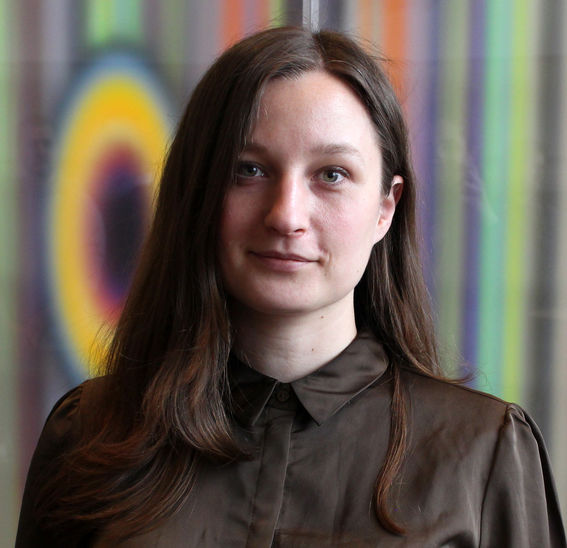
CEST researchers Hilda Sandström and Patrick Rinke, along with collaborators from Aalto University, the University of Helsinki and Tampere University, conducted a comprehensive review of the current state of data-driven compound identification in atmospheric mass spectrometry. This perspective article outlines crucial steps required from the atmospheric chemistry community to implement the identification of compounds using modern smart algorithms.
Despite the acknowledged complexity and sheer number of potential atmospheric organic compounds, detailed knowledge of their reaction mechanisms, intermediates, and products is lacking. Efforts to gain new fundamental knowledge about these atmospheric processes persist, primarily relying on mass spectrometry. However, existing experimental data libraries and manual identification methods struggle to cope with the shear number, large variability and complexity inherent in atmospheric compounds and processes.
While smart compound identification algorithms have demonstrated state-of-the-art performance in other chemical disciplines, their implementation in atmospheric chemistry has been hindered by the scarcity of training data from such atmospheric mass spectrometry studies. The researchers have provided examples of how these machine learning-based compound identification tools could be effectively utilized in conjunction with soft ionization techniques commonly employed in atmospheric mass spectrometry.
Establishing automated and improved identification methods for atmospheric compounds is pivotal to advance our basic understanding of atmospheric chemistry. Crucially, the paper proposes an action plan to create an infrastructure for development of data-driven compound identification in atmospheric mass spectrometry. Following this initial review, Sandström and collaborators now aim to initiate the development and testing of these future intelligent identification methods to help identify atmospheric compounds.
The perspective article was published in Advanced Science under DOI: 10.1002/advs.202306235.
For more details contact
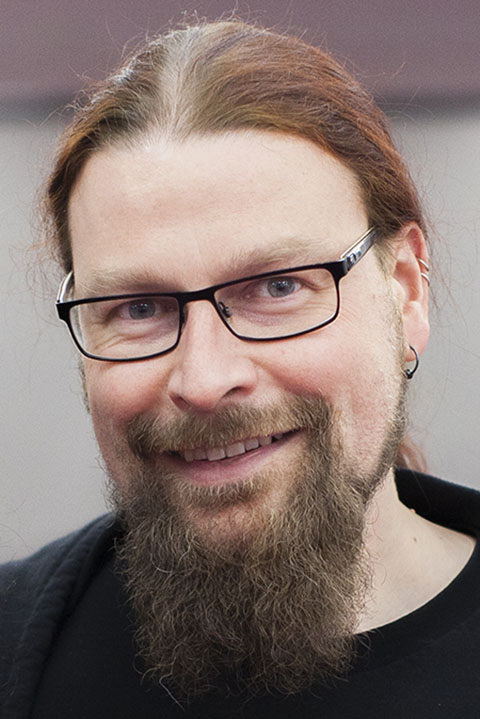
Read more news

DeployAI Partners Gather for Heart Beat Meeting in Helsinki
The European DeployAI project's partners gathered for the Heart Beat meeting hosted by Aalto University Executive Education in Helsinki.
Get to know us: Associate Professor Maria Sammalkorpi
Sammalkorpi received her doctorate from Helsinki University of Technology 2004. After her defence, she has worked as a researcher at the Universities of Princeton, Yale and Aalto.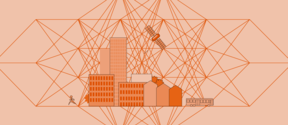
Aalto computer scientists in ICML 2024
Computer scientists in ICML 2024