Can smart devices really understand us?

Digital smart helpers have leaped from Sci-Fi literature into our pockets and on to our tables. Amazon’s Alexa, Apple’s Siri and Google Assistant are taking us away from computer and mobile handset screens – and making the spoken word our new user interface.
It is already easy to check news, choose music, order a cab and command household smart devices using voice control.
But how are such AI-utilising smart helpers in fact able to understand us?
Phase 1: speech recognition
In order to function, smart helpers must always be on. They’ll hibernate and listen to their environment until they recognise a key word uttered within range.
Amazon’s virtual assistant, for example, wake up when it hears its name Alexa. An LED ring on the smart speaker turns blue to indicate that it has awoken.
Apple’s Siri also functions with the same principle. When it hears the prompt Hey, Siri, it starts recording and uploading the user’s speech to recognition software stored on a cloud service.
The digitised speech is first spliced into short bits only fractions of a second in length.
“Everything starts with spectrum analysis, i.e. examining the frequencies found there. Patterns, which describe different sounds, are created in the frequency space,” says Associate Professor Mikko Kurimo from Aalto University’s Department of Signal Processing and Acoustics.
All material redundant from the perspective of speech recognition, such as the pitch of the speaker’s voice and background sounds, is removed in conjunction with splicing.
“In other words, it tries to find patterns that indicate what speech sounds have been uttered,” Kurimo says.
Speech recognition is made more difficult by that fact that we speak incoherently, swallow words and use gestures and utterances. The words we speak can also sound alike, as is the case with, for example ate and eight.
“These days, speech recognition is more and more often performed with deep neural networks,” Kurimo says.
Deep neural networks mimic the way the brain operates and consist of certain types of simple calculators known as artificial neurons. A neural network becomes efficient when interconnected neuron layers communicate with the neurons of the same and the next layer.
In addition to statistical sound models, neural network speech recognition search algorithms utilise language models built with the help of extensive text materials. Language models predict the probability a word will occur after another word as well as the likely way in which it will be pronounced. This helps weed out unlikely words to speed up recognition.
“A speech recognition application thus performs the task of finding the sentence the user most probably spoke,” Kurimo says.
Phase 2: processing natural language
The aim of natural language processing is to decipher the meaning of text – i.e. identify what the user wants from its digital helper.
Neural networks are also utilised in natural language processing. Speech data is scoured automatically for key words and phrases in order to ascertain what the user’s words might possibly relate to.
Neural networks are trained for their tasks by feeding them a large volume of data for processing and then comparing their output values to known correct values. Corrections are made until the result no longer improves. After this, the system is capable of operating independently.
One project headed by Kurimo has researched the production of automatic descriptions of audiovisual material. Among other things, archived Yle videos were chosen as source material. The developed method is able to simultaneously interpret both the speech recorded on the video as well as the moving video image – and can generate a text description of them. The system was taught by using human-written descriptions of the same videos as points of reference.
The size of the databases used to teach deep neural networks is a central factor. This is why commercial digital helpers are being produced by giant corporations like Amazon, Apple, Google and Microsoft.
“Major companies have access to extensive databases, and they can perform automation quite easily. It arduous to start making a chatbot from scratch. You have to accumulate a database somehow.”
Phase 3: fulfilling the request
The last phase is to fulfil the user’s request. In addition to information retrieved from the net, digital helpers take advantage of, for example, the contact details, location information and calendar on the user’s phone in order to form a better idea of what the user wants.
This is why a digital helper can appear surprisingly smart when fulfilling simple requests like connecting a call, looking up weather information or ordering a pizza.
But ask a helper like this to tell you what’s going on in Silicon Valley, and it will provide a clumsy answer containing random search results related to the term Silicon Valley. A digital helper would be unable to deduce whether it is being asked about the history, weather or companies active in the area.
“They run out of smarts the moment you go beyond their design space,” Mikko Kurimo says.
There has also been a shift to employing deep neural networks in generating voices for digital helpers. Speech sounds are always interconnected in natural speech, and incompatible sounds were precisely what made early smart helpers sound so robotic. Today, neural networks perform calculations on the fly to enable the correct pronunciation of the phrases spoken in reply.
“A synthetic speech generator is fed the syllables and words to be emphasised as input, and these make the speech sound natural. The generated signal is then transmitted to the user’s terminal device for playback.”
Towards individualised user interfaces
Even the most conversational AI will not, for quite some time, be able to serve as a worthy debate partner like we’ve seen in so many science fiction movies.
Professor Antti Oulasvirta from the Department of Communications and Networking considers it problematic for voice user interfaces that they are unable to actually understand language.
“AI doesn’t learn language sort of by engaging in physical and social interaction. It cannot learn the linguistic frame of reference to which words or gestures refer to.”
Research on the interaction between humans and AI-employing systems is nevertheless progressing all the time, and the area of possible application is expanding in tandem. One such application area is using computational models to improve user interfaces, a subject that Oulasvirta’s research group has been studying.
For example, a user’s browsing history can be used to reformat a website to a layout that feels immediately familiar to the user.
“It is possible to create a more pleasant browsing experience in this way. Headers, for example, could almost always be found in the same spot.”
Fresh research subjects have also been discovered within an activity as mundane as inputting text. Coupling cognitive science, a field that researches phenomena related to observation, learning and memory, with AI enables the building of models, which accurately predict how a person’s individual characteristics affect, for example, writing on a smartphone display.
When such models are connected with a machine optimiser that simulates alternatives, the user interface can be tailored to suit a specific user. This process has identified smartphone use solutions for older people who suffer from shaky hands, for example.
Oulasvirta’s team has also created a new layout for French computer keyboards, which was recently approved by the standardisation authority of France.
“All of France will be typing special characters in a way determined with the aid of our optimiser,” he says.
A research project dealing with the modeling of emotions is also ongoing.
“In the final analysis, the field of AI deals with presenting human matters computationally,” Oulasvirta says.
He points out that the familiar journey planner found on smartphones is also based on AI, even though most users would not think of it as an AI application. Oulasvirta’s view on the matter is, however, clear.
“Whenever some intellectual capacity can be realised computationally, it, in my opinion, represents AI.”
Text: Panu Räty. Illustration: Ida-Maria Wikström.
This article is published in the Aalto University Magazine issue 23 (issuu.com) October 2018.
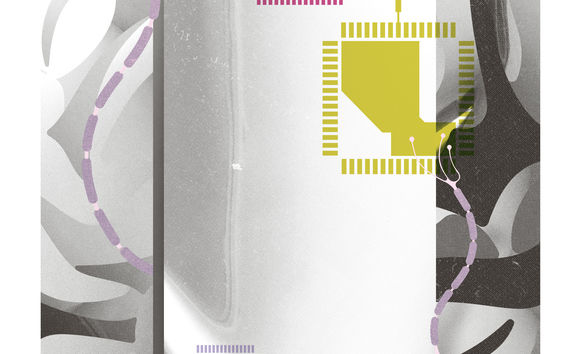
Fresh research subjects have also been discovered within an activity as mundane as inputting text. Coupling cognitive science, a field that researches phenomena related to observation, learning and memory, with AI enables the building of models, which accurately predict how a person’s individual characteristics affect, for example, writing on a smartphone display.
A brief AI glossary
- Algorithm
Definition of how to perform a task, which opens the details of the process step by step.
The term program refers to the expression of an algorithm in a programming language. - Artificial intelligence
Refers to a computer’s ability to engage in intelligent activities.
Is a broad and fluid umbrella concept for various inference principles. - Applied (narrow/weak) artificial intelligence
The only type of AI humanity has produced so far. Produces solutions for a predetermined application area by combining analytics and automation. - Artificial general intelligence
The idea of mimicking human intelligence on computers made popular in science fiction literature. Sometimes an AGI is presented as becoming sentient. Our technology has not even come close to building an AGI for now. - Chatbot (bot)
A software robot that performs tasks automatically mimicking human interaction. The most sophisticated versions are AI-based collections of programs that can search for spoken or text answers on limited subject matters. - Machine learning
Methods by which computers can learn to reach a desired outcome independently based on examples and experience. - Neural network
A machine learning architecture and algorithms, which have some loose inspiration in how human brains function. They consist of interconnected artificial neurons i.e. simple processors. Adding layers to a neural network deepens it and enables the performance of increasingly complex tasks.
Compiled by Panu Räty.
- Published:
- Updated:
Read more news

DeployAI Partners Gather for Heart Beat Meeting in Helsinki
The European DeployAI project's partners gathered for the Heart Beat meeting hosted by Aalto University Executive Education in Helsinki.
Get to know us: Associate Professor Maria Sammalkorpi
Sammalkorpi received her doctorate from Helsinki University of Technology 2004. After her defence, she has worked as a researcher at the Universities of Princeton, Yale and Aalto.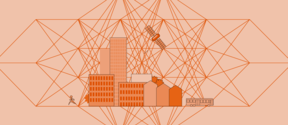
Aalto computer scientists in ICML 2024
Computer scientists in ICML 2024