Department of Computer Science: MSc Thesis Presentation
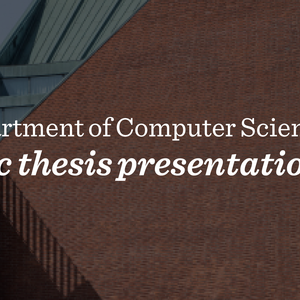
When
Where
Event language(s)
A Critical Look at the Identifiability of Causal Effects with Deep Latent Variable Models
Author: Severi Rissanen
Supervisor: Pekka Marttinen
Date: Friday 30 April 2021
Time: 12:00
Zoom: https://aalto.zoom.us/j/65372372126
Abstract
Causal inference, dealing with the questions of when and how we can make causal statements based on observational data, has been a topic of growing interest in the deep learning community recently. On the one hand, causal inference promises to provide traditional machine learning and AI with methods for explainability, domain adaptation, and causal reasoning capabilities in general. On the other hand, many deep learning methods for improving causal inference have been proposed.
One active line of research has studied the use of deep latent variable models (DLVM) to address the important problem of unobserved confounding in causal models. While they have yielded promising results and theory exists on the identifiability of some simple model formulations, we also know that causal effects cannot be identified in general with latent variables. The aim of this thesis was to investigate this gap between theory and empirical results, using the causal effect variational autoencoder (CEVAE) as a case study.
To gain understanding on the identifiability of causal effects with CEVAE, we run extensive experiments under multiple synthetic and real-world data sets and provide some theoretical results. While CEVAE seems to work reliably under some simple scenarios, it does not identify the correct causal effect with a misspecified latent variable or a complex data distribution, as opposed to the original goals of the model. Our results show that the question of identifiability cannot be disregarded, and we argue that more attention should be paid to it in future work.