Defence of doctoral thesis, Homayun Afrabandpey
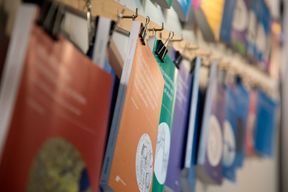
When
Where
Event language(s)
Title of the doctoral thesis is "Methods for Probabilistic Modeling of Knowledge Elicitation for Improving Machine Learning Predictions"
Datasets with a small number of samples and a large number of variables are common in many real-world applications. Statistical modeling in such applications is challenging and it is known that models have limits in how low in sample size they can go. An approach to alleviate these challenges is to rely on other sources of information to be combined with the modeling to improve the final model. One of these additional sources is the domain expert; a person who knows the application of interest well enough. However, extracting knowledge from human expert is difficult.
This dissertation developed approaches to efficiently extract knowledge from human users and effectively combine them with machine learning models through Bayesian framework to improve the predictive performance of models. Among different types of knowledge, this thesis work focused on knowledge on variables, in particular (i) relevance of single variables to a task at hand, and (ii) pairwise similarities of variables. The conclusion from this study is that in cases where the training data contains small number of samples with large number variables, if modeled properly, expert knowledge on features can bring predictive gain for the model comparative to collecting more samples.
Literature is replete with various approaches for extracting knowledge from expert and combining them with the model. However, the literature mostly focused on extracting knowledge on samples. The approaches developed in this dissertation are complementary to those approaches in the literature and the combination of these methods can be adopted in applications with small number of samples and large number of variables to improve modeling.
Opponent: Professor Patrick Shafto, Rutgers University, USA
Custos: Professor Samuel Kaski, Aalto University School of Science, Department of Computer Science
Contact information of the doctoral candidate: Homayun Afrabandpey, Nokia, [email protected]
The defence will be organised remotely, via online platform (Zoom). Link to the defence
Zoom Quick Guide (www.aalto.fi)
The doctoral thesis is publicly displayed 10 days before the defence on the publication archive of Aalto university (aaltodoc.aalto.fi).
Electronic doctoral thesis (aaltodoc.aalto.fi)
- Published:
- Updated: