CEST - Research: Theory and method development

People involved: Marc Dvorak, Dorothea Golze
We mainly work with quantum mechanical first principles. For the atomic and electronic structure we use density-functional theory (DFT) and for excited states (theoretical spectroscopy) Green's function methods, such as the GW approach. On the DFT side, we actively develop hybrid density-functionals that mitigate self-interaction errors, while staying computationally tractable. On the Green's function side, we are investigating approaches that go beyond GW such as second-order screened exchange self-energies. We also seek the connection between DFT and many-body Green's functions theory to devise advanced density-functionals, for example functionals that lead us beyond the random-phase approximation (RPA).
Some of our current projects are:
Core level spectroscopy is a powerful tool to characterize molecules, liquids and adsorption processes at surfaces. Accurate computational methods to predict core excitations are important for the interpretation of experimental results. However, the reliable computation of core levels remains a challenge. We are developing new approaches to predict core level spectra for molecular systems.
Strongly-correlated electrons are those which cannot be described by independent particle methods like DFT. Besides their importance to fundamental physics, strongly-correlated materials are becoming increasingly relevant for technological applications. We are researching new approaches to strong correlation that combine the best features of many different theories.
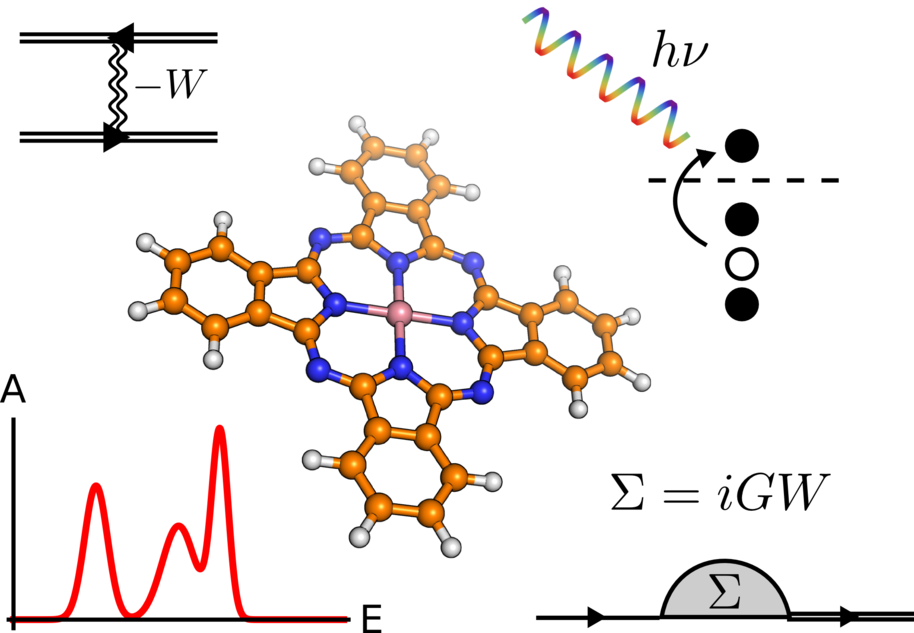

For wide dissemination, we implement most of our developments into the Fritz Haber Institute ab initio molecular simulations package (FHI-aims). If you are interested in using FHI-aims for your own work or if you would like to contribute to FHI-aims, please contact us.
More on machine learning methods is given on the Machine Learning page.
Related publications:
Piecewise linearity in the GW approximation for accurate quasiparticle energy predictions, M. Dauth, F. Caruso, S. Kümmel, and P. Rinke, Phys. Rev. B 93, 121115(R) (2016)
Random-phase approximation and its applications in computational chemistry and materials science, X. Ren, P. Rinke, C. Joas, and M. Scheffler, J. Mat. Sci 47, 7447 (2012)
- Published:
- Updated: